Clustered matrix approximations: Test 6
Rectangular matrix A, with dense non-diagonal block structure, and independent row and column clustering
Contents
- Load data
- Cluster rows of a rectangular matrix (bipartite graph)
- Cluster columns of a rectangular matrix (bipartite graph)
- Determine dense blocks
- Fraction of non-zeros within dense blocks
- Compute clustered matrix approximatons
- Compute relative error in % of clustered matrix approximation
- Compute cosines of principal angles
- Compute SVDs of all dense blocks
Load data
clear % Load processed NotreDame data load NotreDame_actors_preprocessed [m,n] = size(A);
Cluster rows of a rectangular matrix (bipartite graph)
% Normalize rows of A B = normalizeColumns(A')'; B = B*B'; % Determine threshold value %[rr,cc,vv] = find(B); %semilogy(sort(vv,'descend')) %grid on % Threshold matrix and cluster with GRACLUS B = matrixThreshold(B,0.1); rnoc = 15; [rprt,~] = graclus(B,rnoc); [rind,rprtsz,rprtc] = reorderVertices(rprt);
---------------------------------------------------------------------- Graclus 1.2 Copyright 2008, Brian Kulis and Yuqiang Guan Graph Information: #Vertices: 81823, #Edges: 1836035, #Clusters: 15 #local search steps: 0 Normalized-Cut... Cut value: 2.434641, Balance: 1.39 Timing Information: I/O: 0.018 Clustering: 4.405 (Graclus time) Total: 4.449 ----------------------------------------------------------------------
Cluster columns of a rectangular matrix (bipartite graph)
% Normalize columns of A B = normalizeColumns(A); B = B'*B; % Determine threshold value %[rr,cc,vv] = find(B); %semilogy(sort(vv,'descend')) %grid on % Threshold matrix and cluster with GRACLUS B = matrixThreshold(B,0.1); cnoc = 20; [cprt,~] = graclus(B,cnoc); [cind,cprtsz,cprtc] = reorderVertices(cprt); spy(A(rind,cind))
---------------------------------------------------------------------- Graclus 1.2 Copyright 2008, Brian Kulis and Yuqiang Guan Graph Information: #Vertices: 94003, #Edges: 6926728, #Clusters: 20 #local search steps: 0 Normalized-Cut... Cut value: 4.299469, Balance: 2.84 Timing Information: I/O: 0.068 Clustering: 15.295 (Graclus time) Total: 15.437 ----------------------------------------------------------------------
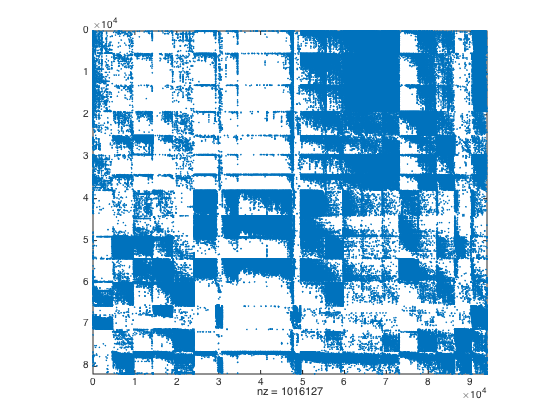
Determine dense blocks
threshold = 0.25; [msk,~,totFrc] = determineDenseBlocks(A,rprtc,cprtc,threshold); clf spy(msk) nDenseBlocks = nnz(msk); disp(['Total number of dense blocks: ', int2str(sum(sum(msk))), ' of ',... int2str(rnoc*cnoc)])
Total number of dense blocks: 73 of 300
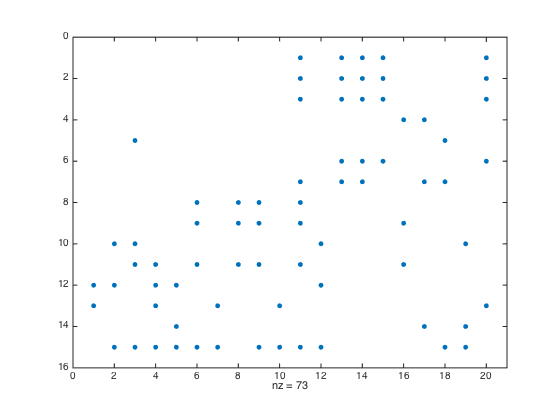
Fraction of non-zeros within dense blocks
[nnzBlks, nnzBlFr] = compClustNnzRect(A,rprtc,cprtc); bar3custom(msk.*nnzBlFr) title(['Fraction of non-zeros in blocks A_{ij}, ', num2str(totFrc), ... ' % in dense blocks '])
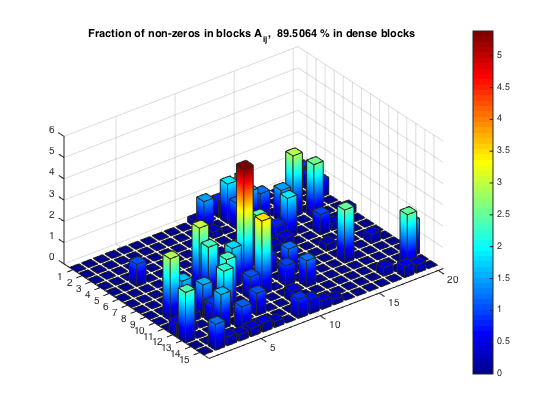
Compute clustered matrix approximatons
% Specify rank k for approximations of dense blocks % Or use different ranks for different blocks k = 15; K = (ones(size(msk))*k).*msk; % Compute approximations of dense blocks of a rectangular matrix [U,S,V] = clustSvdsRect(A,K,rprtc,cprtc,'svds'); % if lansvd is available and prefered %[U,S,V] = clustSvdsRect(A,rprtc,cprtc,k,'lansvd'); % Specify parameters for clustered randomized algorithms p = 2; P = (ones(size(msk))*p).*msk; q = 2; Q = (ones(size(msk))*q).*msk; [Ur,Sr,Vr] = clustRandAlgRect(A,K,P,Q,rprtc,cprtc); SS = cell2mat(S); SSr = cell2mat(Sr);
Compute relative error in % of clustered matrix approximation
nA = norm(A,'fro'); relerrCmapp = sqrt( nA^2 - norm(SS, 'fro')^2 )/nA*100; relerrCmappR = sqrt( nA^2 - norm(SSr,'fro')^2 )/nA*100; % Compute memory consumption memCmapp = memUsageCmapp(U,SS,V,'non-sym'); memCmappR = memUsageCmapp(Ur,SSr,Vr,'non-sym'); % Get rank k for a regular matrix approximation with the same memory usage [m,n] = size(A); [memRmapp,kreg] = memUsageRmapp(m,n,memCmapp,'sym'); [Us,Ss,Vs] = svds(A,kreg); relerrRmapp = sqrt( nA^2 - norm(Ss,'fro')^2 )/nA*100; % fpns = floating point numbers disp('-------------------------------------------------------------------') fprintf('Clustered matrix approximation: relErr = %10.2f %% \n', relerrCmapp) fprintf('Clustered matrix approximation: relErr = %10.2f %% (randomized) \n', relerrCmappR) fprintf('Regular matrix approximation: relErr = %10.2f %% \n', relerrRmapp) disp('-------------------------------------------------------------------') fprintf('Clustered matrix approximation: memUsage = %10.0f fpns \n', memCmapp) fprintf('Clustered matrix approximation: memUsage = %10.0f fpns (randomized) \n', memCmappR) fprintf('Regular matrix approximation: memUsage = %10.0f fpns \n', memRmapp) disp('-------------------------------------------------------------------')
------------------------------------------------------------------- Clustered matrix approximation: relErr = 94.50 % Clustered matrix approximation: relErr = 94.65 % (randomized) Regular matrix approximation: relErr = 97.43 % ------------------------------------------------------------------- Clustered matrix approximation: memUsage = 12637035 fpns Clustered matrix approximation: memUsage = 14503159 fpns (randomized) Regular matrix approximation: memUsage = 12682720 fpns -------------------------------------------------------------------
Compute cosines of principal angles
% Compute angles for column space matrices angCol = compSubspaceAngles(Us,U,rprtc); % Compute angles for row space matrices angRow = compSubspaceAngles(Vs,V,cprtc); plot(angCol) hold on plot(angRow) grid on hold off title('Cosines of principal angles between clustered and regular subspace matrices') legend('Column space matrices','Row space matrices','location','SouthWest') xlim([1,kreg])
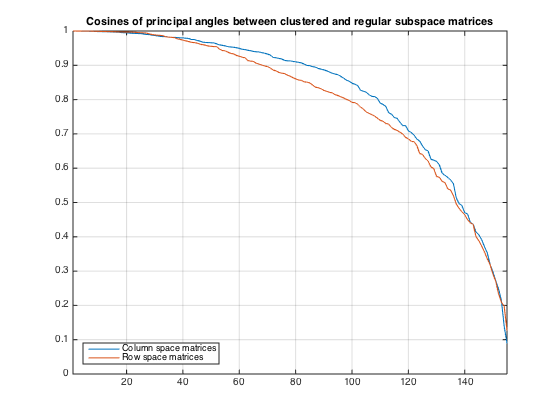
Compute SVDs of all dense blocks
[UU,SS,VV] = clustSvdsExtRectCell(A,K,rprtc,cprtc,'svds'); % Plot singular values for dense blocks clf for i = 1:rnoc for j = 1:cnoc if K(i,j) > 0 plot(diag(SS{i,j}),'-x'); hold on end end end grid on hold off
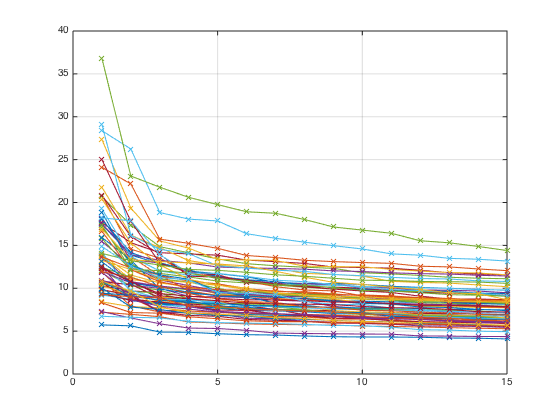